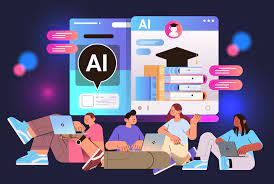
OpenAI showcases a control methodology for superintelligent AI.The researchers instructed GPT-2 to direct the significantly more potent GPT-4.
In the future, it is anticipated that we will develop AI systems surpassing human intellectual capabilities. This could be highly beneficial if these systems help solve complex issues like cancer or climate change. However, it could pose a significant risk if these AIs act in ways contrary to human interests, and we lack the intelligence to control them.
To address this concern, OpenAI initiated its superalignment program earlier this year. This ambitious initiative aims to discover technical methods for controlling or “aligning” superintelligent AI systems with human goals. OpenAI is allocating 20 percent of its computational resources to this project and aims to devise solutions by 2027.
The primary challenge lies in dealing with a future problem related to models that are yet to be designed, and for which we lack access. Collin Burns, a member of OpenAI’s superalignment team, acknowledges the complexity of studying such a problem but emphasizes the necessity of doing so.
The initial preprint paper from the superalignment team outlines one approach they explored to overcome this challenge. Rather than assessing if a human could effectively supervise a superintelligent AI, they tested a weaker AI model’s capability to supervise a more powerful one. In this instance, GPT-2 was assigned the task of supervising the considerably more potent GPT-4. The contrast in power is substantial, with GPT-2 having 1.5 billion parameters, while GPT-4 is rumored to boast a staggering 1.76 trillion parameters (though OpenAI has not officially disclosed the figures for the more advanced model).
Jacob Hilton from the Alignment Research Center, a former OpenAI employee not associated with the current research, finds the approach intriguing. He notes that creating effective empirical testbeds for aligning the behavior of superhuman AI systems has been a persistent challenge. According to Hilton, the presented paper takes a promising step in addressing this issue, and he expresses anticipation about the future developments it may lead to.
“This is a challenge that pertains to future models, models we haven’t yet figured out how to design, and models we currently lack access to.”
The OpenAI team assigned three types of tasks to the GPT pair: chess puzzles, a suite of natural language processing (NLP) benchmarks involving commonsense reasoning, and questions based on a dataset of ChatGPT responses. In the ChatGPT task, the objective was to predict the preferred response among multiple options chosen by human users. GPT-2 underwent specific training for each task, but due to its limited size and capabilities, its performance was suboptimal. Subsequently, the training was transferred to a version of GPT-4 with only basic training and no fine-tuning for these specific tasks. It is crucial to note that even with basic training, GPT-4 outstrips GPT-2 in terms of capabilities.
The researchers sought to determine whether GPT-4, under the guidance of its weaker supervisor, GPT-2, would make similar mistakes. Surprisingly, the more robust model consistently outperformed its less capable supervisor. GPT-4 excelled, particularly in the NLP tasks, achieving accuracy levels comparable to GPT-3.5. While its performance on the other two tasks was less impressive, the results were encouraging enough to motivate the team to persist in refining these tasks. This phenomenon is labeled weak-to-strong generalization, suggesting that the powerful model possessed implicit knowledge of task execution, even when provided with subpar instructions.
The team observed that this approach yielded the best results with NLP tasks due to their relatively straightforward nature with clear right and wrong answers. Conversely, it performed less effectively on tasks involving the ChatGPT database, where determining preferred human responses was more nuanced. As Leopold Aschenbrenner, a researcher on the superalignment team, explains, some responses were subtly better or worse, contributing to the challenges encountered in this aspect of the experiment.
Is it feasible for this alignment technique to extend to superintelligent AI?
Burns provides an illustration of how a similar scenario might unfold in a future era with superintelligent AI. If tasked with coding, the AI might generate a million lines of highly complex code that interacts in entirely novel ways beyond human programming conventions. This could pose a challenge in discerning whether the AI is truly executing the intended task. To address potential risks, humans may supplement the coding instruction with a precautionary directive, such as avoiding catastrophic harm during the coding process. If the model has absorbed the concept of weak-to-strong generalization, it might possess an understanding of what constitutes catastrophic harm and be more adept than its human supervisors in assessing the safety of its work.
Burns emphasizes the limitations of human supervision, stating that we can only oversee simple examples within our comprehension. For superintelligent models to generalize to more complex examples, they must understand safety considerations and whether adherence to instructions is crucial, aspects challenging to directly supervise.
While some may interpret these findings as a drawback for superalignment, Burns argues that a superintelligent AI following incorrect instructions is undesirable. Moreover, in practical scenarios, weak supervisors may struggle with exceedingly challenging problems, requiring a superintelligent entity to discern the correct solutions.
In a move to encourage further exploration of such challenges, OpenAI has announced a $10 million grant initiative for research on diverse alignment approaches. Pavel Izmailov, another member of the superalignment team, highlights the shift from alignment being primarily theoretical to becoming a focus for academics, graduate students, and the wider machine learning community. The grants include specific provisions for graduate students, offering a $75,000 stipend and a $75,000 compute budget.
Burns expresses enthusiasm about the initiative, emphasizing that it presents an opportunity to empirically address the alignment problem with future superhuman models. While acknowledging that it’s a challenge for the future, he stresses the importance of making tangible progress in the present through iterative empirical efforts.